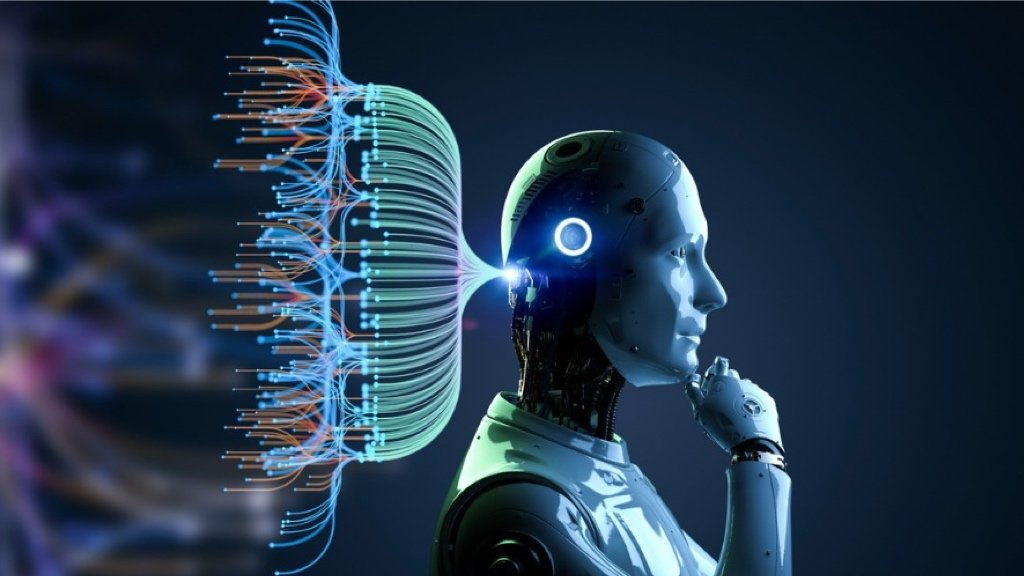
Stalled Disruption: How "Messy" Workflows Are Protecting Human Jobs from AI
Artificial intelligence has shown remarkable progress in tackling tasks once thought beyond its reach, such as passing challenging graduate-level exams or writing at a professional standard. This remarkable capability raises a paradox: if AI can handle such complex work, why haven't we seen it replace vast numbers of human jobs?
What This Article Covers
In the pages to come, we'll explore new research on how AI struggles with "messy" tasks, even as it excels at well-defined, linear workflows. We'll also look at real-world cases of job displacement, discuss timelines for broader disruption, and outline practical strategies for adapting to a future driven by AI.
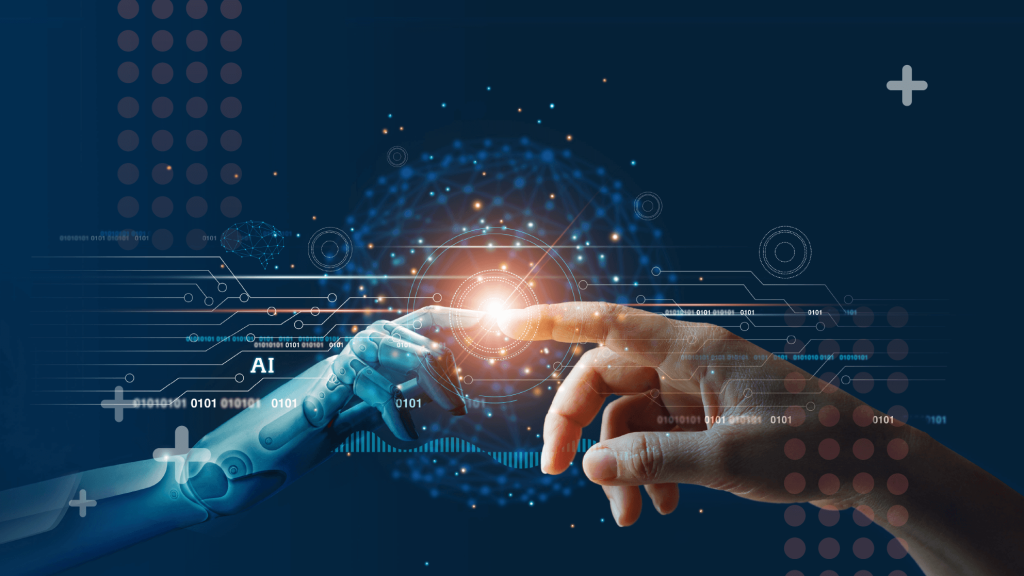
The AI Paradox: High Capability, Low Disruption
Artificial intelligence tools—particularly large language models (LLMs)—have demonstrated an ability to perform sophisticated tasks that many experts once deemed too complex for automation. From passing law-school entrance exams to generating human-like essays, these breakthroughs reveal an impressive capacity to mimic and sometimes surpass human performance in narrowly defined contexts.
Yet, despite this comprehensive proficiency, we do not see a massive wave of unemployment in roles that involve similar levels of cognitive complexity. This discrepancy highlights a fundamental puzzle: if AI can tackle exams at an Ivy League level, why isn’t it replacing more knowledge-intensive jobs at a faster pace?
Why the Disruption Stalls
One reason for this seemingly slow disruption is that AI’s current strengths often centre on structured, predictable tasks. While it can excel at providing well-formed answers to specific queries, it struggles with unstructured work that requires constant adaptation and real-time decision-making—a far cry from the tidy environments of standardised tests.
Moreover, “messy” responsibilities in many jobs involve context switching, ambiguity, and dynamic interaction with people. In these situations, AI’s lack of flexible reasoning becomes more evident. Humans can rely on emotional intelligence, implicit knowledge, and reading social cues—skills that remain challenging for even the most advanced models.
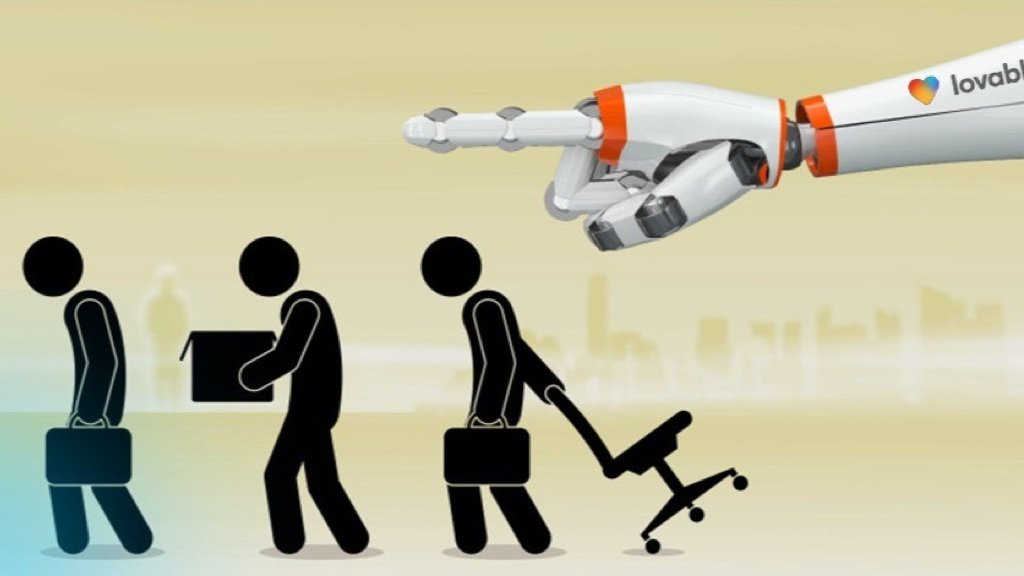
Real-World Impact: Who’s (Truly) Feeling the Strain?
Dropping Employment for Writers and Developers
Recent employment data reveals an unexpected twist: instead of typical office roles like travel agents or bookkeeping clerks taking the hit, it’s writers and software developers who seem most affected. Industry snapshots show declining job numbers for these occupations, diverging sharply from their usual growth trends in the past couple of years.
One reason is that writing and coding can be broken into structured, discrete tasks that AI handles exceptionally well - whether it’s drafting product descriptions or debugging lines of code. For freelancers or contractors in these fields, businesses can seamlessly replace human labour with AI tools without extensive bureaucratic hurdles.
Why Them?
These roles align so closely with AI’s core competencies—linear, goal-defined workflows - that entire projects can be automated from start to finish. A marketing agency needing quick copy, or a startup requiring repetitive coding, may see immediate cost-saving benefits by switching to AI-driven solutions.
Moreover, high rates of freelancing in writing and coding exacerbate this phenomenon. If an organisation can pay per project rather than maintain a salaried position, swapping in AI is a relatively low-risk move, prompting a faster shift than in more entrenched, full-time roles.
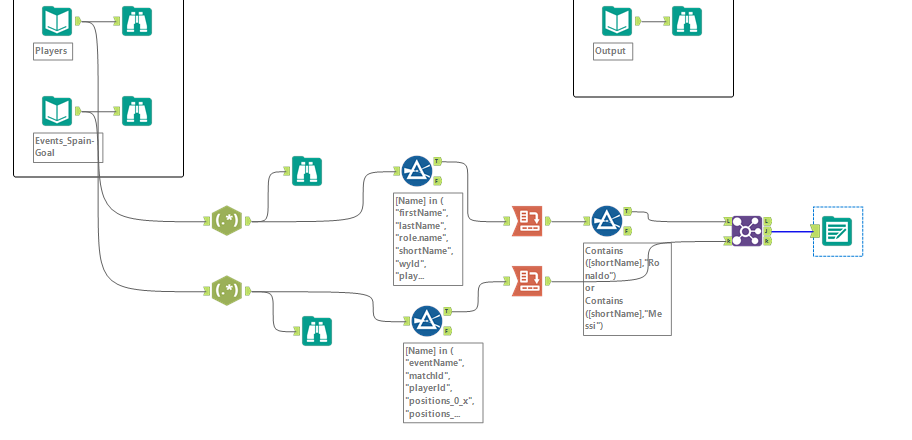
The Protective Power of ‘Messiness’
Despite the impressive capabilities of modern AI, many of its breakthroughs rely on predictable input-output relationships. In reality, human jobs frequently involve unstructured workflows characterised by shifting contexts, ambiguous goals, and unpredictable interactions.
While an LLM can easily draft a memo or respond to a standard query, it often struggles with multifaceted reasoning - juggling rapid updates, empathising with end users, and making judgment calls on the fly.
Take, for instance, an executive assistant scheduling last-minute meetings with stakeholders in different time zones. Coordinating these moving parts requires interpreting vague or conflicting preferences, resolving unforeseen scheduling conflicts, and reading subtle social cues. E
ach of these tasks demands a level of adaptability that cutting-edge AI, for all its computational horsepower, is still learning to master. Until models can thrive amid these messy, human-centric nuances, human labour remains the linchpin in roles that prioritise agility.
Case Study Example 1: Admin Work in Healthcare
An emerging AI startup deployed a model to handle patient inquiries at a large healthcare network. The system excelled at providing scripted responses on insurance coverage and appointment availability - until patients deviated from the expected questions, adding personal details about symptoms or emotional concerns. Because the
AI was not designed to handle compassionate dialogue or triage more complex cases, calls were frequently escalated back to human agents. This outcome underscores how unstructured, back-and-forth communication reveals AI’s current limitations in real-world contexts.
Case Study Example 2: Cross-Department Coordination
Meanwhile, a multinational logistics firm tested a chatbot to manage inter-departmental shipping requests. Although the bot could generate routine shipping labels and track deliveries, issues arose when it had to negotiate priority changes or integrate last-minute legal documents from separate teams.
In these scenarios, managers needed to step in and clarify ambiguous goals - something the AI could not do on its own. The pilot ended with a hybrid workflow: humans handled all unexpected escalations and strategic decisions, while the bot continued to perform well-defined tasks like updating status fields and emailing automated confirmations.
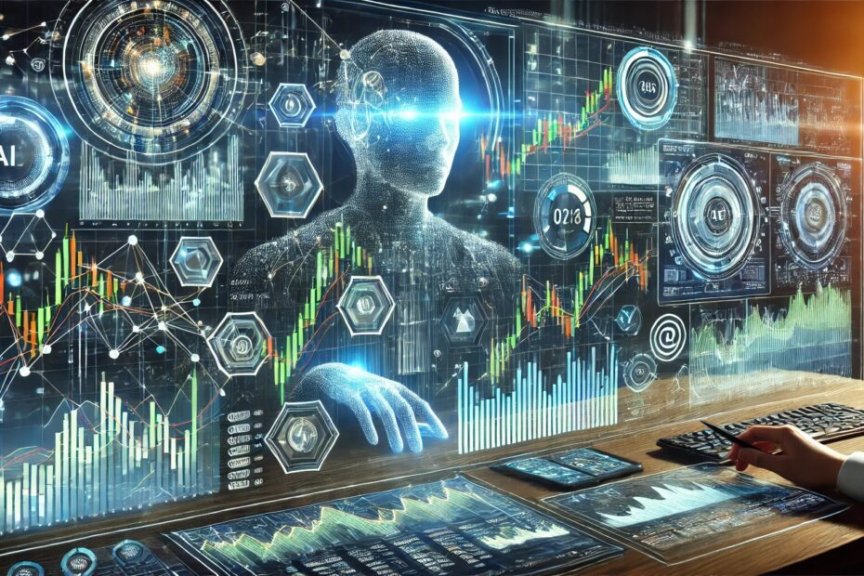
The Timeline: How Soon Before Widespread Displacement?
While current AI models may struggle with unpredictable or multitasking scenarios, they are rapidly improving. Recent advancements in reinforcement learning and context-aware architectures suggest a trajectory that could soon extend AI’s reach into tasks we once believed immune.
As research labs iterate on more versatile frameworks—capable of tracking multiple objectives and adapting on the fly - today’s limitations may give way to next-generation systems that handle a broader array of real-world complexities.
Experts caution that this progress should not be underestimated. Each iterative leap has historically been faster and more far-reaching than many in the field predicted. From improved language comprehension to more effective decision-making, AI’s evolution has a compounding effect: the better it gets, the more it accelerates further improvements. Consequently, roles once safeguarded by messy workflows may soon face a genuine threat.
Historic Comparisons and Expert Predictions
Looking at previous automation waves can offer perspective. When industrial machines first threatened manual labour in the 19th and early 20th centuries, societies adapted over decades, not years.
However, the digital revolution progressed far more swiftly, upending sectors such as manufacturing, customer service, and finance within a single generation. Many analysts argue AI represents a similarly transformative technology - potentially reshaping white-collar work at a much faster pace than earlier shifts.
Meanwhile, futurists remain divided on the exact timeline, with some predicting a gradual takeover and others forecasting a rapid displacement once certain technical thresholds are met.
In either case, increased collaboration between AI developers, economists, and policymakers will be pivotal, ensuring that society prepares for - and meaningfully shapes—the next seismic shift in the labour market.
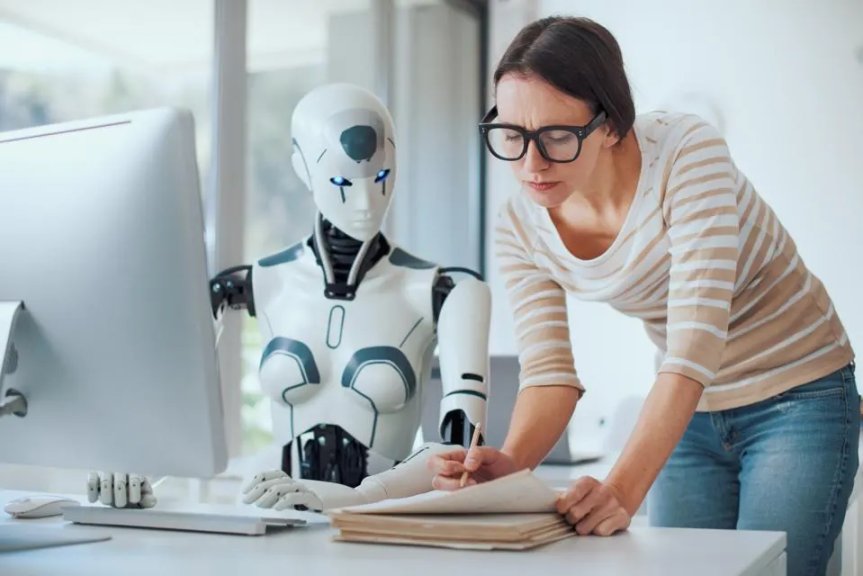
Adjusting to an AI-Driven Future
As AI begins to take over tasks once thought secure, the best approach for professionals is to develop complementary skills. Creativity, empathy, and domain expertise become especially crucial, as these traits remain largely beyond what AI can achieve.
Identify Complementary Skill Sets
For example, a marketing professional who uses AI to draft content while incorporating personalised storytelling will continue to be sought after. Similarly, a project manager skilled in interpreting vague goals, managing stakeholder relationships, and integrating human inputs can leverage AI's efficiency without being replaced.
Additionally, as generative models and machine learning systems advance, a new range of AI-related careers is emerging. Roles such as prompt engineering, model oversight, and ethical auditing demonstrate how human judgment remains essential for responsible and effective AI use.
Focus on Complex, Value-Added Roles
Instead of waiting for technology to catch up, workers can actively shift towards responsibilities that rely on holistic thinking, interpersonal communication, or strategic planning—areas where AI typically falls short. For instance, employees who learn to interpret AI outputs with critical understanding and adapt them for nuanced audiences offer significant value compared to those whose roles focus solely on linear tasks.
Likewise, organisational leaders need to think beyond mere cost-cutting. Investing in upskilling teams, fostering an innovation mindset, and actively monitoring AI advancements can prevent a company from being caught off-guard. By cultivating environments where human and AI collaboration is standard, businesses can grow alongside the technology without facing major disruptions.
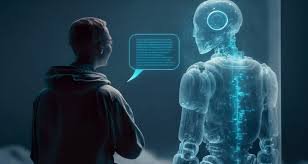
Generative AI’s remarkable ability to deliver high-level work on demand hasn’t yet caused the massive job disruptions many feared. Instead, these new technologies show their greatest impact where tasks are straightforward and predictable, such as writing and coding.
Meanwhile, roles that revolve around unstructured, unpredictable workflows - coordinating multiple parties, handling nuanced conversations, and adapting to fluid requirements - still hold firm against the AI wave.
However, as model architectures become more sophisticated, and as researchers refine techniques to help AI manage complex inputs, today’s safe havens may not remain so for long. The so-called “messiness” that protects these roles today could prove only a temporary shield against the advancing capabilities of machine intelligence.
Looking Ahead
Ultimately, adapting to AI means embracing a hybrid future. Workers and organizations that skillfully integrate AI tools into their workflows, while cultivating human-centric expertise such as empathy, creative thinking, and strategic judgment, will be best positioned for success.
Rather than an outright replacement, AI offers a collaborative partnership that can boost productivity - but only for those prepared to capitalize on its strengths while mitigating its weaknesses.